Mahnaz Akbari Kasten
Bio:
Mahnaz has degrees in Math, Computer Science, and Data Science. She’s worked in different IT roles over past two decades. She was gravitated towards data related projects, and got her second master degree in that are. Now, she’s working in a startup, Contact Center Tools, as data scientist working on challenging NLP problems. Fighting inequality has been her mission for almost entire life. She founded Seattle Women in Data Science (SeaWiDS) group a little over a year ago in order to shed light on the important role of women in this field across all industries. She also believes that having a group like SeaWiDS in the community will encourage younger generation of female professionals to consider data science field. The group has been very active since then. Monthly talks, study groups, mentoring sessions, and interview preparation group are some of the activities from SeaWiDS. The community of SeaWiDS has over 1000 members, and they keep building connections with each other and with other organizations who support their mission..
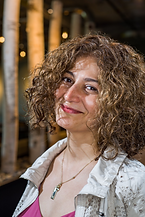
Shivani Patel
Bio:
Shivani is a Data Scientist at SAP Concur where she works on developing machine learning algorithms for the ExpenseIt Product. She holds two bachelor’s degrees (speech and hearing sciences and math), as well as a master’s in statistics from Oregon State University. Shivani is passionate about equity in public education and she a member of the Renton Schools Foundation Board where she focuses on supporting an equitable curriculum of elementary STEM education. Shivani believes that movements like WiDS are invaluable in supporting the career development of women in technology which is why she is a Regional Ambassador for the WiDS conference.
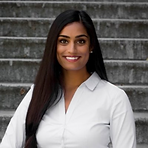
Loida Erhard
Bio:
Loida is a program officer with PATH’s Health Systems and Analytics team. She is a public health systems researcher with eight years of experience in academic and applied research with a focus on monitoring and evaluation of public programs. In her current role, she supports program and research design, implementation, and complex multi-sectoral partner coordination. She holds a Master of Public Health from Emory University and a B.S. from the University of Washington in Biology. Loida is passionate about supporting evidenced-based policy making and providing technical support on identifying and evaluating effective social programs.
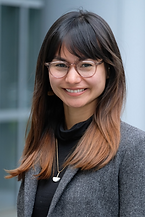
Kate Farris
Bio:
Kate Farris is a Data Scientist and Security Engineer. She holds a PhD from the Thayer School of Engineering at Dartmouth as well as a Bachelor’s of Science degree in Mathematics from the University of Arizona. Kate previously served as an officer in the United States Air Force. She has contributed to research that spans the areas of computer security, computational data analysis, and visualization. She is most well known for her empirical research in cyber-security vulnerability management. Kate currently works at Microsoft as a Security Technical Program Manager where she is building up a program in intelligent automation of cyber security detections for the areas of Security Assurance and Incident Response.
​
Abstract:
Cyber-Security is a vast and complex domain. The challenges are so great that there are typically never enough resources to address all problems. Further, taking more principled approaches to how these problems are defined, solved and measured as successful or not will bring much needed advances to the discipline. As the security landscape is constantly changing with attacker and defender behaviors displaying non-stationary behavior, it becomes a very difficult to apply traditional machine learning and statistical models in the automation pipelines. This talk will review some of those challenges, as well as present opportunities within this discipline to drive a more principled approach in how we think about cyber-security data, and how it can be used to quantify and measure some of cyber-security’s most pressing challenges.
​
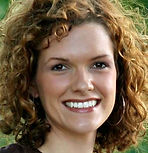
Rebecca Grollman
Bio:
Rebecca Grollman is a data scientist at Bsquare where she works with companies deploying IoT initiatives to help them understand their data capabilities and build actionable predictive models using machine learning. She also researches and builds tools to accelerate data science processes and provide deeper insights. Rebecca holds a BA in physics and anthropology from Ithaca College as well as an MS and PhD in physics from Oregon State University.
​
Abstract:
Traditional (reactive) maintenance is expensive and data utilization is very low in the transportation industry. As transportation companies begin to collect more data, they are turning to predictive maintenance to improve their bottom line. I will present a case study where I helped a transportation company transition to incorporate predictive maintenance into their routine. The story begins with collaborating with multiple subject matter experts to decide on a feasible use-case. From there, I built a machine learning model to predict the probability that a specific part needed to be replaced on a vehicle in 3 days. I will also discuss the next steps moving forward to integrate this model and future models into their organization.

Wendy Grus
Bio:
Wendy Grus is currently a senior data analyst at Hulu in Seattle where she helps product and development teams make data-driven decisions based on user behavior. Before moving over to tech, she worked in biotech as a bioinformatics analyst/scientist. She volunteers for organizations that empower women and girls in music and technology, such as Rain City Rock Camp and Seattle PyLadies.
​
Abstract:
My team at Hulu makes an operational intelligence tool that is used internally to create realtime reports to monitor app health and feature usage. While existing telemetry tells us the who and when of its usage, it does not tell us why people use it. To gain insights into how this tool is used by product and tech, I built a flask app to mine JIRA data for references to its reports using the Python jira library.
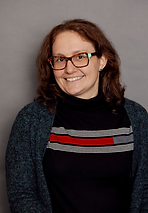
Jill Larson
Bio:
Jill is a seasoned leader with experience across the full product lifecycle - from research to product development, branding, GTM, demand generation, customer advocacy and sales. She has accumulated 20+ years of experience and has a track record of understanding business problems and solving them in order to grow the bottom line. Jill has a deep understanding of product creation and marketing in software, hardware and FSI products.
.png)
Mengyuan Liu
Bio:
Mengyuan is a data scientist at SAP Concur where she works primarily on building machine learning engines to support one of the company’s core products: ExpenseIt, an app that allows automatic recognition of key fields in receipt images. Before joining Concur, Mengyuan obtained PhD in bioengineering from University of Washington, specializing in applying machine learning and computer vision technologies to medical imaging.
​
​
Abstract:
The data science team at SAP Concur is responsible for the machine learning infrastructure for two products: ExpenseIt and ML Audit.These two products are at very different stages: ML Audit is a project which is at its initial stage where we building automation for an existing manual audit product, while ExpenseIt is a more mature product which is being pushed to more international markets. In this talk, two data scientists working on these two products will talk about the specific challenges faced during two different stages of the products.
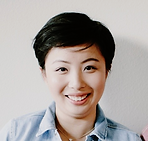
Catherine Nelson
Bio:
Catherine Nelson is a Senior Data Scientist for Concur Labs at SAP Concur, where she explores innovative ways to use machine learning to improve the experience of a business traveller. She is particularly interested in privacy-preserving ML and applying deep learning to enterprise data. In her previous career as a geophysicist she studied ancient volcanoes and explored for oil in Greenland. Catherine has a PhD in geophysics from Durham University and a Masters of Earth Sciences from Oxford University.
​
Abstract:
Data privacy is a huge topic right now for any business using personal data. People are questioning whether they should allow companies to collect data about them, and they are asking about what happens to that data after they hand it over. Machine learning systems often depend on data collected from their users to make accurate predictions. But can we build cool products powered by machine learning while still providing privacy for our users? In this talk, I’ll discuss some practical options for increasing privacy, from simple methods to differential privacy, and show some examples of how we can incorporate these into products. I’ll show how we’ve built a Data Washing Machine that allows us to provide nuanced levels of privacy via a simple API. I’ll also explore the tradeoff between user privacy and model accuracy, and show how we can retain model accuracy even when privacy is increased.
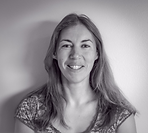
Jacqueline Noils
Bio:
Dr. Jacqueline Nolis is a Principal Data Scientist at Nolis, LLC: data science and AI consulting firm started by her and Heather Nolis. Jacqueline has over a decade of experience doing data science in industry, working with companies ranging from DSW and Union Bank to Microsoft and Airbnb. Her academic research covered optimization under uncertainty with a specialization in electric vehicle routing. In her free time, Jacqueline enjoys using programming to make jokes, including creating the viral website tweetmashup.com
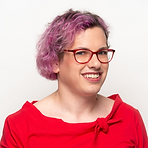
Peggy Shao
Bio:
Peggy Shao joined Airbnb Seattle last May as a data scientist in the support product team. She leverages machine learning to provide better customer support for the Airbnb community through more efficient routing. Prior to her current role, she developed large scale credit risk models in Capital One Auto Finance. Peggy holds a master in Statistics from Rice University, and is excited to meet more WiDS in PNW after moving to Seattle last year.
​
Abstract:
Do you wonder what problems Airbnb Seattle is solving for the company? What machine learning product do we build to support the Airbnb community? What challenges and tools does Airbnb have for machine learning? Join us and find out answers to these questions and more.
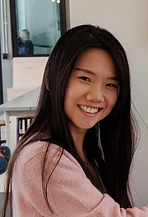
Suchitra Sundararaman
Bio:
Suchitra is a Data Scientist at SAP Concur working primarily on building the machine learning framework for the ML Audit product. ML Audit is a project which will help automate the existing audit tasks and scale the traditional audit service by leveraging various Machine learning techniques. Suchitra interned as BI analyst at Concur last year and transitioned to this role as she was very passionate about Data Science. She has Masters degree from University of Washington where she specialized in Data Science and Business Intelligence.
​
Abstract:
The data science team at SAP Concur is responsible for the machine learning infrastructure for two products: ExpenseIt and ML Audit.These two products are at very different stages: ML Audit is a project which is at its initial stage where we building automation for an existing manual audit product, while ExpenseIt is a more mature product which is being pushed to more international markets. In this talk, two data scientists working on these two products will talk about the specific challenges faced during two different stages of the products.

Tina Tang
Bio:
Tina Tang is global product marketing lead at SAP for machine learning and esports & advanced analytics. She is co-founder of Womeninbigdata.org, a 9000+ strong volunteer organization spanning 5 continents. Tina also serves as board member of MinasList.org and advises startups and non-profits. With 20 years experience in the technology industry, she has held diverse roles including strategy, sales, communications, operations, partner, community, documentation, and special projects. She holds degrees from the University of Texas at Austin. Tina lives in Palo Alto, California with her husband, cat, and 2 donkeys.
​
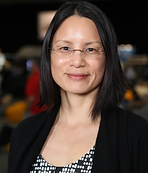
Amelia Taylor
Bio:
Dr. Amelia Taylor is a data scientist at Zymergen, a technology company integrating machine learning and manufacturing technologies to create novel products and materials from biology. At Zymergen, she builds data science tools to more efficiently design experiments, collect and store data, and analyze that data to accelerate the pace of Zymergen’s engineering cycles. Prior to joining Zymergen, Taylor competed for and completed the Insight Data Science Fellows Program, a rapid immersion training program where, in just four weeks, she created an end-to-end data tool for a utility company to predict which of their meters had been tampered with. Before becoming a data scientist, she spent 16 years as a mathematics professor, including at Colorado College.
​
Abstract:
At Zymergen we integrate robotics, software and biology to provide predictability and reliability to the process of rapidly improving microbial strains through genetic engineering. One critical part of this process is rapid, robust and useful processing of data to provide scientists with the information they need to make the next round of changes and decide which strains to promote. In particular, our robots allow us to run hundreds of experiments in parallel and our analytical automation allows us to clean and process those data in near real time. A first step is to identify outliers that arise in the data due to multiple opportunities for process failure and with this comes both the challenge of modeling outliers, and the problem of model evaluation for both selecting a model and tuning parameters. In Robots, Biology and Unsupervised Model Selection, I present an end-to-end approach, in python, for model selection, with an emphasis on parameter tuning, for unsupervised outlier detection algorithms. This problem is well studied for supervised and even semi-supervised (labels are human evaluation) anomaly and outlier detection algorithms, but there are few resources readily available when it comes to unsupervised algorithms in this arena.

Tian Tian
Bio:
Tian works as a research engineer in Qualtrics, Seattle. She developed many NLP models that enable Qualtrics to show the market that our technology is advanced and leading. Before joining Qualtrics, she worked as a research assistant at CMU and helped the team win LoReHLT16. She also has publications in COLING, SIGIR, ANT and Journal of Machine Translation.
​
Abstract:
Technical approaches to generating high-quality unsupervised topic models for short text. In Qualtrics, the average comment is only about 100 characters long, yet Qualtrics customers expect and require actionable topics to be automatically generated for their document sets. Due to sparse word co-occurrences, traditional topic models work poorly on short texts. Recent work aggregates short texts to augment word co-occurrence. In this session we will discuss technical approaches to emphasize relevant information and improve topic coherence. We will also discuss incorporating externally well-trained word embeddings to introduce contextual semantic information and phrase models and a knowledge-based ranking models to discover phrase-level topics and inter-topic rankings, respectively.
